Artificial Intelligence (AI) has revolutionized how we interact with technology, enabling machines to perform tasks once impossible without human, manual intervention. Among the various branches of AI are Generative AI and Predictive AI, two powerful paradigms, each with unique goals and applications in numerous industries. This article will delve into these two types of AI, highlighting their definitions, key features, techniques, applications, and differences.
What is Generative AI?
Generative AI (GenAI) is a technology that enables the creation of new content by learning from already existing data sets. While traditional AI systems classify or analyze data, generative models create new instances using machine learning. The content produced by GenAI can be text, image, sound, code, or video. Among the most popular tools currently used in GenAI is OpenAI’s GPT-3 language model, which generates text from prompts, DALL-E, which creates images, and Tabnine, which is a coding assistant. The most important feature of the content generated with GenAI, however, is that it is deceptively similar to that created by humans.
Key Features of Generative AI
1. Creativity and Originality:
- GenAI can produce content that is not copies of input data, but new creations drawn from learned sources. Such a capability allows it to genuinely mimic human creativity in numerous fields, like art, music, or literature or the production of new software for which code is necessary.
2. Data Augmentation:
- GenAI can be used to create new synthetic data points that are then added to existing data sets using AI algorithms in machine learning. This process is used to improve the performance and accuracy of models as much as possible with the help of increasing the amount of training data.
3. Interactivity:
- GenAI-based interactive tools can be freely used by users without technological experience. Chatbots and apps allow simple prompts and commands, so any user, regardless of their level of technological knowledge, will be able to generate the content they need.
Techniques Used in Generative AI
1. Generative Adversarial Networks (GANs):
- GAN networks consist of two competing neural networks – a generator that creates outputs and a discriminator that evaluates them. Through iterative training, the generator improves its outputs to the point where they become indistinguishable from real data. An example of GANs technology is FaceApp, which modifies the entered photos in a very realistic way.
2. Variational Autoencoders (VAEs):
- VAEs consist of an encoder and a decoder, and their task is to recognize features or patterns from the input data automatically. In this process, input data is taken and then processed by a neural network to generate new data like that on which it was trained. This technology is used to produce realistic images and 3D organ modeling in medicine or scientific research to analyze large data sets and discover their patterns and relationships.
3. Large Language Models (LLMs):
- LLMs are advanced AI models that can understand and generate text in a way that resembles human language. They are trained in massive data sets, allowing them to acquire extensive knowledge. They operate on complex neural networks to recognize subtle differences in language, and their flexibility allows them to be used in a wide range of fields – from customer service (chatbots) to coding and developer support (GitHub Copilot). You can read more about LLMs here.
Applications of Generative AI
1. Pharmaceutical Industry:
- GenAI supports drug discovery by analyzing large data sets like genomic and phenotypic information. Advanced machine learning models make it possible to predict which genes are most influential in causing diseases. Another use of AI algorithms is also to simulate interactions between a drug and biological targets – this helps determine whether a treatment will be effective. But what’s most fascinating, GenAI revolutionizes drug discovery by designing novel molecular structures, predicting their interactions and biological targets. This accelerates the identification of promising drug candidates for further development.
2. Finance Industry:
- GenAI improves risk assessment and fraud detection in finance by analyzing transactional patterns to identify anomalies. Using historical data, these models can generate predictive inferences and forecasts from which companies can mitigate risks. Repetitive manual work can also be reduced by using GenAI to create automated financial reports or analyze market trends to construct effective strategies. Read more about GenAI in Business Intelligence.
3. Public Sector:
- GenAI improves public services and government operations by automating document generation, using chatbots on government websites to serve citizens, or supporting predictive analytics for resource allocation and crisis management.
Techniques Used in Predictive AI
1. Regression Analysis:
- This statistical method is used to predict the value of a continuous variable based on other variables (called independent variables). Regression models are trained on historical data to identify patterns among them, which can then be used to predict performance. This method is used in financial forecasting, evaluating the effectiveness of a campaign, or predicting demand among a product’s audience.
2. Time Series Forecasting:
- One valuable data analysis technique is time series forecasting, which involves predicting future values and performance based on historical data that has been collected at regular intervals. Such a process involves analyzing the data’s patterns, trends, and seasonality, enabling more accurate predictions about future events.
3. Machine Learning Algorithms:
- Decision trees, random forests, or neural networks are AI techniques for creating predictive models. They learn from historical data and continuously improve accuracy, making them valuable tools for predicting future industry trends and performance.
Applications of Predictive AI
Finance:
Banks use predictive analytics for credit scoring, fraud detection, and risk assessment by analyzing transaction patterns and customer behavior.
Healthcare:
Predictive models can forecast disease outbreaks or patient readmission rates by analyzing historical patient data and demographic information.
Public Sector:
Predictive analytics can be used to anticipate social needs, such as demand for public services, enabling better resource planning and more efficient management.
Difference Between Generative AI and Predictive AI – A Comparative Analysis
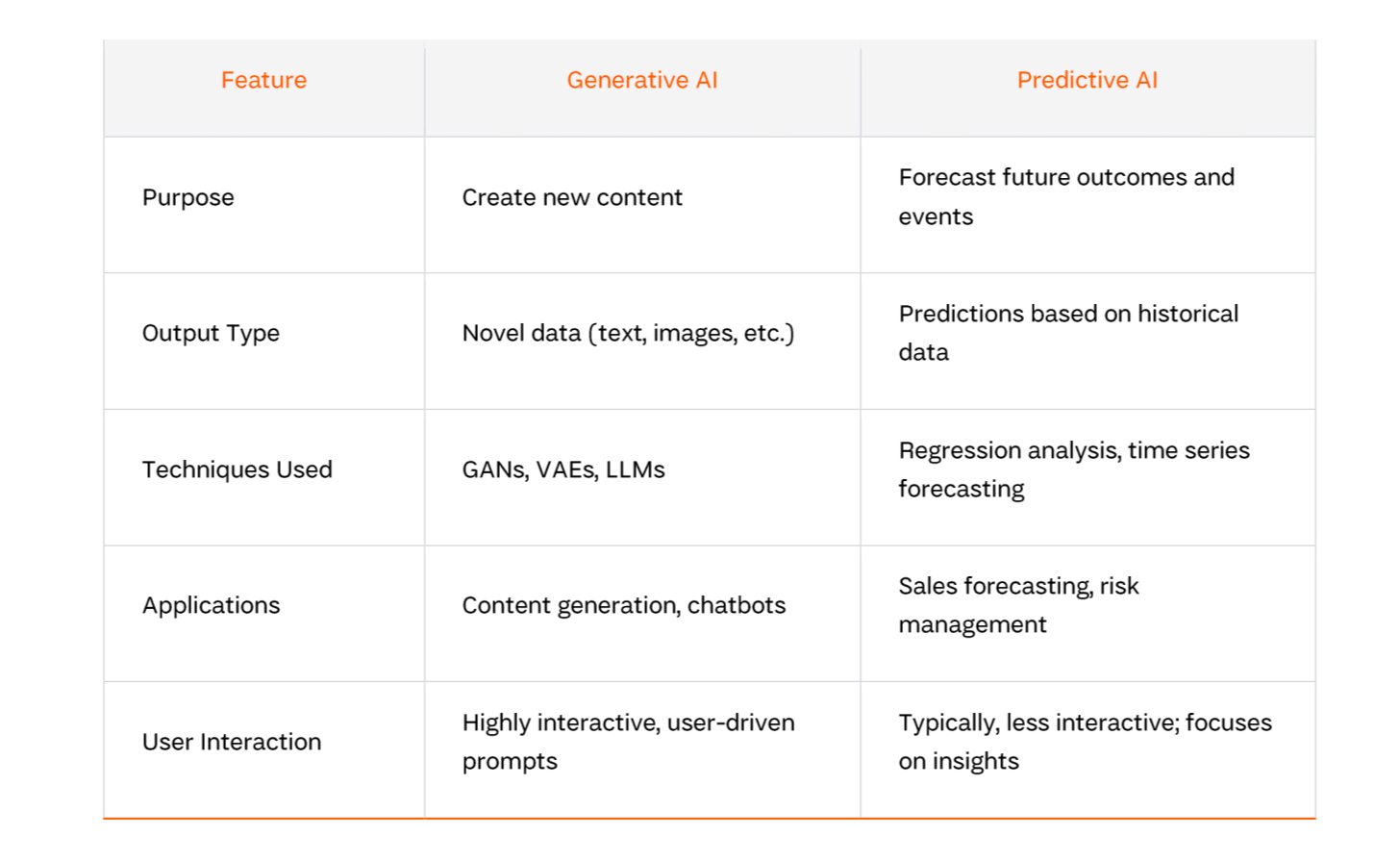
Both generative and predictive artificial intelligence present significant advances in AI, the effects we see daily in numerous tools and uses. While Generative AI finds application in generating new content that improves workflow and influences higher creativity in multiple fields, Predictive AI is a source of precious information that allows companies to make more informed and accurate decisions based on historical data.
Understanding these differences between the two branches of AI will allow you to choose the right approach for your specific business needs – from creating engaging customer content to effectively predicting future trends or avoiding risks.
Integrating these two powerful branches of AI will undoubtedly bring opportunities for innovation across industries and using the potential of generative or predictive AI is already becoming crucial for companies that want to remain competitive in the digital world. Check out how we at Billennium can tailor suitable AI models and solutions for your business.